
- This event has passed.
Suvrajeet Sen – USC
October 29, 2018 @ 3:30 pm - 4:30 pm
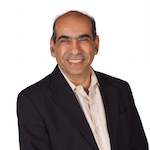
Bio: Professor Sen served as a program director at NSF where he was responsible for the Operations Research, and the Service Enterprise Engineering programs. At NSF, he also headed the Cyber infrastructure planning activities of the Engineering Directorate. Concurrently with his appointment at NSF, he was a professor of Systems and Industrial Engineering at the University of Arizona.
Professor Sen has served on the editorial board of several journals, including Operations Research as Area Editor for Optimization, and as Associate Editor in INFORMS Journal on Computing, Telecommunications Systems, as well as Operations Research. He is the past-Chair of the INFORMS Telecommunications Section and founded the INFORMS Optimization Section. Professor Sen is a Fellow of INFORMS.