
- This event has passed.
Divya Singhvi — Learning Personalized Product Recommendations with Customer Disengagement
January 9, 2020 @ 11:00 am - 12:30 pm
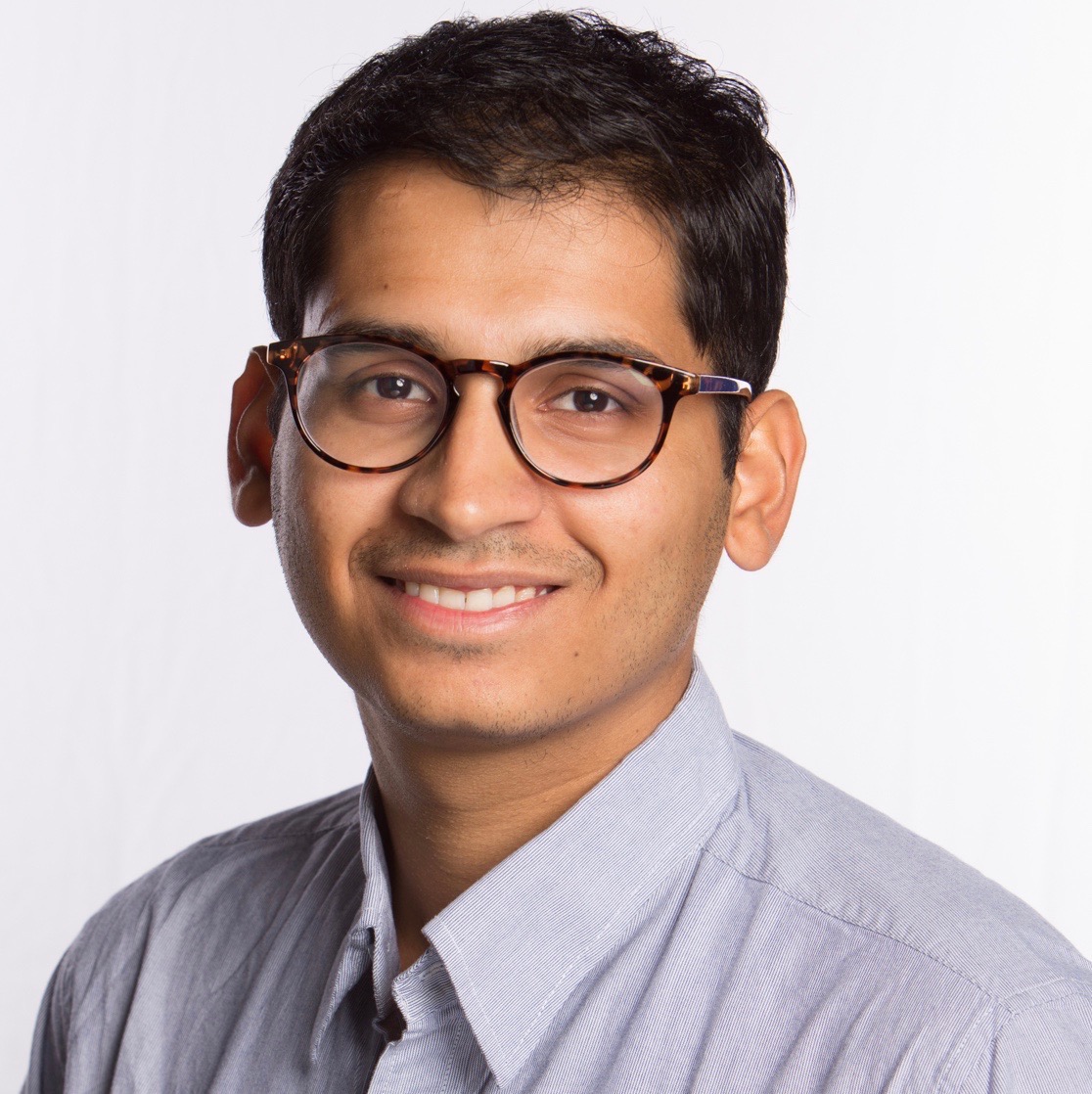
Problem definition: We consider the problem of personalized product recommendations on platforms when customers have unknown preferences. Importantly, customers may disengage from the platform when offered poor recommendations.
Academic / Practical Relevance: Online platforms are increasingly learning customer preferences and personalizing recommendations using bandit algorithms, which balance an exploration-exploitation trade off. However, customer disengagement—a salient feature of platforms in practice—introduces a novel challenge to this setting, since exploration may cause customers to abandon the platform. We propose a novel learning algorithm that constrains exploration to achieve better performance.
Methodology: We present evidence of customer disengagement using a sequence of ad campaigns from a major airline carrier; this motivates our model of customer disengagement, where a customer may abandon the platform when offered recommendations that are irrelevant to her. We formulate the customer preference learning problem as a linear bandit, with the notable difference that the customer’s horizon length is a function of past recommendations.
Results: We prove that any algorithm in this setting achieves linear regret. Thus, no algorithm can keep all customers engaged; however, we can hope to keep a subset of customers engaged. Unfortunately, we find that classical bandit learning as well as greedy algorithms provably over-explore, thereby incurring linear regret for every customer. We propose modifying bandit learning strategies by constraining the action space upfront using an integer program. We prove that this simple modification allows our algorithm to achieve sublinear regret for a significant fraction of customers.
Managerial Implications: Platforms should be careful to avoid over-exploration when learning customer preferences if customers have a high propensity for disengagement. Numerical experiments on movie recommendations data demonstrate that our algorithm can improve customer engagement by up to 80%.
Divya Singhvi is a fifth-year doctoral candidate at the Operations Research Center at MIT, advised by Professor Georgia Perakis. His research lies at the intersection of machine learning and operations management. He has worked on problems related to optimal demand learning, pricing, recommendations and logistics in online and offline retail operations.