
- This event has passed.
IEOR Seminar Series: Shipra Agrawal, Columbia University
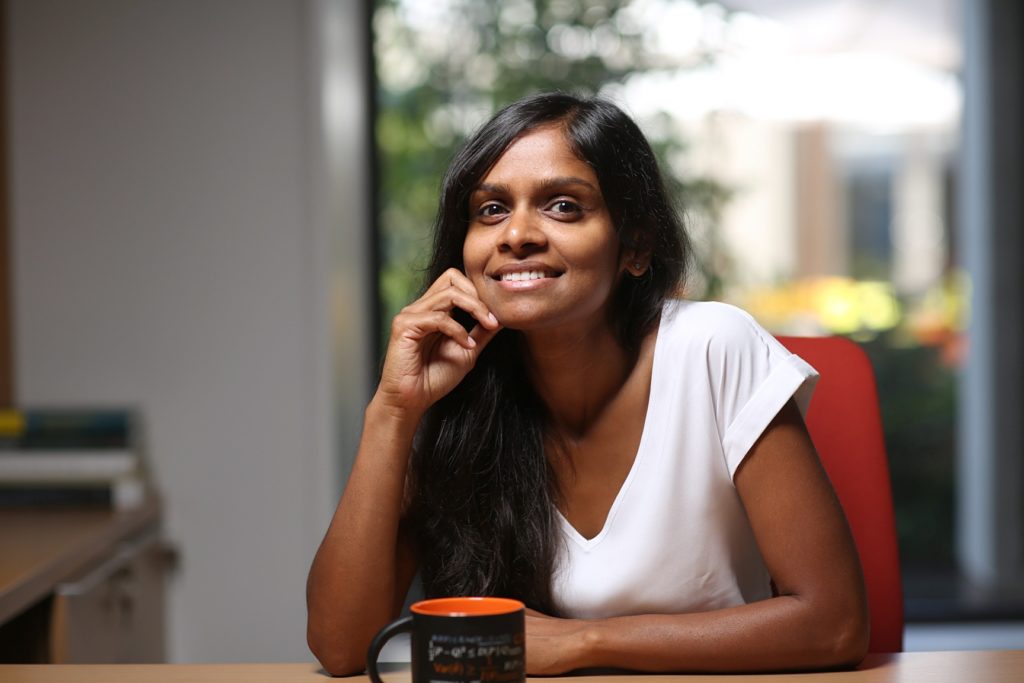
Talk Title: Dynamic Pricing and Learning under the Bass Model
Abstract: In this talk I will discuss a novel formulation of the dynamic pricing and demand learning problem, where the evolution of demand in response to posted prices is governed by a stochastic variant of the popular Bass model with parameters (α, β) that are linked to the so-called "innovation" and "imitation" effects. Unlike the more commonly used i.i.d. demand models, in this model the price posted not only affects the demand and the revenue in the current round but also the evolution of demand, and hence the fraction of market potential that can be captured, in future rounds. Finding a revenue-maximizing dynamic pricing policy in this model is non-trivial even when model parameters are known, and requires solving for the optimal non-stationary policy of a continuous-time, continuous-state MDP. In this work, we consider the problem of dynamic pricing in conjunction with learning the model parameters, with the objective of optimizing the cumulative revenues over a given selling horizon. Our main contribution is an algorithm with a regret guarantee of O (m^2/3), where m is mnemonic for the (known) market size. Moreover, we show that no algorithm can incur smaller order of loss by deriving a matching lower bound. We observe that in this problem the market size m, and not the time horizon T, is the fundamental driver of the complexity; our lower bound in fact indicates that for any fixed α,β, most non-trivial instances of the problem have constant T and large m. This insight sets the problem setting considered here uniquely apart from the MAB type formulations typically considered in the learning to price literature.
Authors: Shipra Agrawal (Presenter), Columbia University
Steven Yin (Co-author), Columbia University
Assaf Zeevi (Co-author), Columbia University
Bio: Shipra Agrawal is an Associate Professor of the Department of Industrial Engineering and Operations Research. She is also affiliated with the Department of Computer Science and the Data Science Institute, at Columbia University. Her research spans several areas of optimization and machine learning, including online optimization, multi-armed bandits, online learning, and reinforcement learning. Shipra serves as an associate editor for Management Science, Mathematics of Operations Research, and INFORMS Journal on Optimization. She is a recipient of a Google faculty research award, an Amazon faculty research award, and NSF CAREER award.