New machine learning technique may help prevent blindness for millions of people with diabetes
Diabetic retinopathy (DR) is the most common cause of vision loss among people with diabetes and a leading cause of blindness. Right now, there are an estimated 415 million people suffering from diabetes worldwide, and this number is projected to grow to 642 million by 2040.
Approximately one-third of diabetic patients have DR. While blindness caused by DR is mostly preventable with early detection and treatment, it is difficult to diagnose, and many patients do not get screened due to the disease’s slow progression, the lack of access to eye specialists, or simple unawareness.
Thanks to cutting-edge machine learning techniques developed by IEOR’s Risk Analytics and Data Analysis Research (RADAR) Lab at the University of California, Berkeley, led by Professor Xin Guo, millions of diabetic patients now have a cheaper and more accurate way to screen for eye disease.
“What is really needed if you are thinking about prevention, is to diagnose the cases in-between,” said Guo. “The average consistency of eye specialists to detect a DR is 65 percent, and if you remove the obvious cases, it is closer to 50 percent. Now what do you get with 50 percent? You flip a coin.”
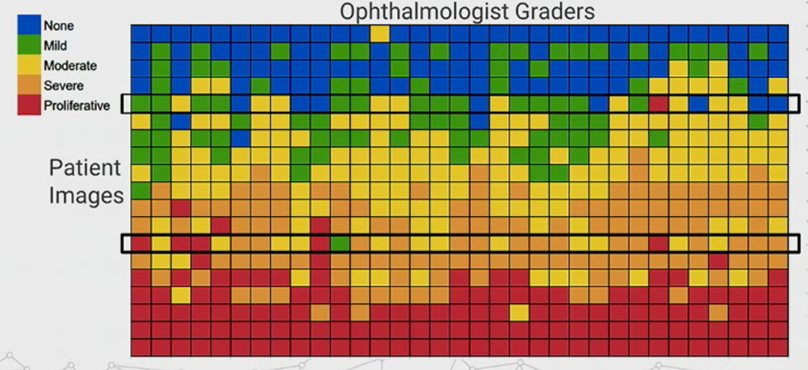
Eye doctors are good at detecting very healthy, and very unhealthy tissue, but have difficulty consistently diagnosing tissue that is in-between.
Guo and her research team from RADAR lab, (including her PhD student Guan Wang and research associate Cheng-ju Wu), thought that perhaps they could develop algorithms using machine learning techniques to assist with diagnoses and help eye specialists improve their consistency. She and her collaborators developed a deep neural network to detect features in retinal images.
But in order to train their algorithm, they would need data that was labeled by expert doctors. They found hospitals in China that were able to provide retinal image data and recruited a team of volunteer doctors to help label the the images as healthy or diseased. Through this network of doctors, each labeling 20-30 images per night, the researchers were able to collect over 100,000 patient cases for their algorithm. And by tracking which patients ultimately got eye disease, they eventually achieved more consistent results than the average eye specialist.
While they have had some success at detecting all seventeen major eye diseases, Guo and her colleagues have been most successful at detecting DR, which the system can now accurately detect about 97 percent of the time. Guo hopes that using the same techniques, she will soon be able to achieve more consistent results with other major eye diseases.
Since their discovery, Guo and her collaborators have installed close to 100 systems in some of the poorest rural areas in China. The villages where the system is implemented do not have consistent access to the internet, and Guo’s algorithm lives on a computer connected to a camera, which gives the doctor the probability that the patient has eye disease, such as 90 percent or 95 percent. So far, Guo estimates that 3 to 5 million people have used these systems since they began their efforts in 2016.
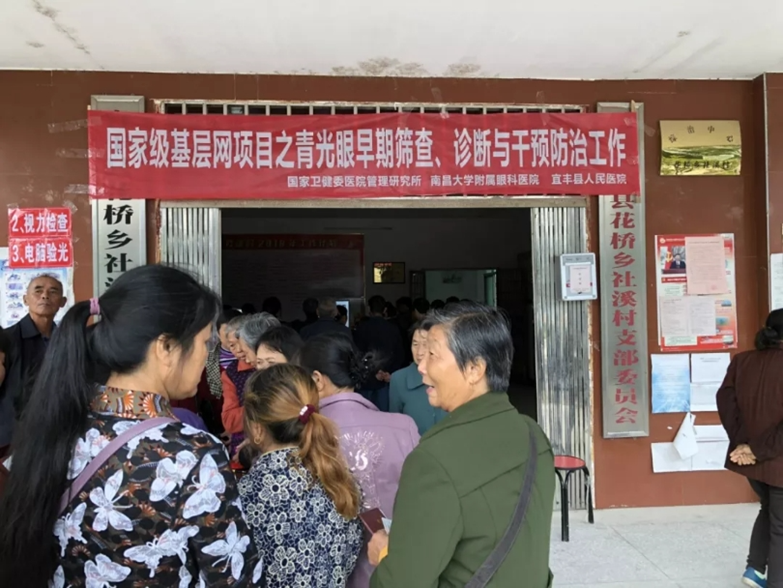
Recently, Guo has started to partner with one of the three major eye hospitals in China. The hospital wants to reach out to more people and Guo is assisting them to create a new remote diagnosis program to detect DR. With a remote diagnosis program, Guo’s algorithm has the potential to help diagnose many more patients.
“We need people and more help because diabetes is a major health problem worldwide,” said Guo. “The major obstacle of diabetic eye disease versus cancer, for example, is that when somebody gets cancer, they get more desperate and they are willing to do almost anything [to get help]. With eye disease, it progresses slowly. So, even though you tell them that the vision loss is irreversible, they can still see now, right? They do not immediately realize that in a year, they may go blind.”