Training Smarter AI: New Research Integrates Human and Machine Feedback
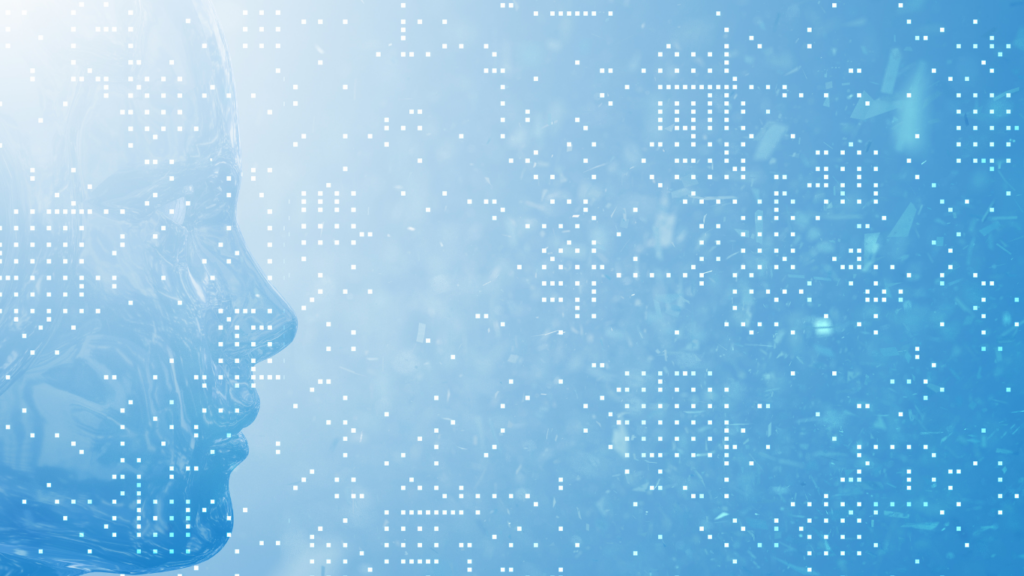
AI models rely heavily on feedback to refine their outputs and meet user needs. While human feedback is highly effective, it is often expensive, time-intensive, and limited by data privacy constraints. For example, training an AI system to detect early signs of cancer in medical scans relies on radiologists to verify predictions and provide annotations. This process is critical but resource-heavy, as it demands expert time and adherence to strict privacy regulations. On the other hand, AI-generated feedback can complement this by employing secondary AI models trained on anonymized imaging datasets to pre-screen scans and highlight potential areas of concern for further review. However, AI-generated feedback may struggle to account for subtle contextual clues or rare conditions that experienced clinicians are trained to detect.
UC Berkeley IEOR researchers have introduced an innovative new approach to training specialized AI models tailored for specific fields. PhD students Haoting Zhang and Jinghai He, along with recent graduate Jingxu Xu and Professor Zeyu Zheng, have developed a simulation optimization framework that combines feedback from humans and AI systems to improve the accuracy and efficiency of these models. Their award-winning paper, Enhancing Language Model with Both Human and Artificial Intelligence Feedback Data, earned the Best Theoretical Paper Award at the 2024 Winter Simulation Conference.
The new simulation optimization framework bridges the gap between human and AI-generated feedback, enabling AI systems to leverage the strengths of both for improved performance. The framework is built around a statistical technique called control variates, which reduces variability during the training process, enabling domain-specific models to better align with human preferences. Additionally, the researchers introduced a resource allocation strategy that prioritizes feedback sources based on cost and quality, optimizing the training process for maximum efficiency.
“Our framework brings together the speed of AI with the depth of human input, enabling more efficient and adaptable training of domain-specific language models,” explained Haoting Zhang, one of the lead researchers.
The potential applications of this research are vast. In healthcare, the framework could streamline processes for developing AI models that analyze sensitive medical data. In finance, it offers a secure way to train systems on private datasets, such as credit risk evaluations or fraud detection. By tailoring these domain-specific models to balance human expertise and AI scalability, Berkeley researchers are setting a new standard for smarter and more efficient AI systems.
With this innovative approach, the Berkeley IEOR team is paving the way for advanced, collaborative AI solutions that address real-world challenges across specialized industries.