New research from Professor Anil Aswani Creates More Inclusive Data-Driven Decision-Making
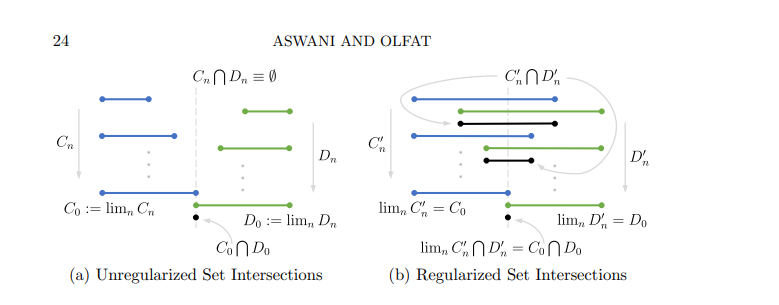
From healthcare to criminal justice and finance to education, powerful algorithms are being developed and applied to all levels of society for decision-making. While AI promises to create data-driven, accurate, and more efficient decisions, algorithms are still capable of making biased predictions.
In recent years policymakers have become increasingly critical of data-driven decision-making due to fears of algorithmic discrimination, which, when left unchecked, can amplify systemic inequities and have dire consequences for communities of color and other vulnerable groups. For example, in October 2019, researchers found that an algorithm used on more than 200 million people in US hospitals to predict which patients would likely need extra medical care was found to favor white patients over black patients heavily. Bias has also been exposed in data-driven hiring decisions, criminal sentencing, credit scoring, and other practices.
With rising concerns about bias, researchers have begun developing data collection and modeling methodologies for fairer algorithms. However, until now, discoveries in algorithmic fairness have been limited in application, with researchers developing statistical techniques that are highly context-based and only applicable in specific, single-use settings. These approaches to fairness often only incorporate numerical attributes, such as income or age, or can only simultaneously accommodate up to two protected groups. These limitations are mainly due to the inherent non-convexity of fairness, making computation notoriously tricky.
In Optimization Hierarchy for Fair Statistical Decision Problems, forthcoming from Annals of Statistics journal, IEOR Professor Anil Aswani and co-author Matt Olfat (PhD IEOR ‘20) develop the first-ever theoretically proven approach to fairness that is not confined to specific, single-use contexts. Using the setting of statistical decision problems, Aswani and Olfat have created a novel method to build linear models that can be applied across numerous diverse groups that represent both numerical and non-numerical characteristics and traits.
Aswani and Olfat's new method allows distinguishing attributes to become stochastically independent variables by characterizing independence as moment conditions. Once moment conditions characterize independence, this allows, them to be incorporated into linear models that can then be numerically computed by solving optimization problems.
With Aswani and Olfat’s novel approach, complex decisions that are often troubled by bias in sectors like health care, criminal justice, and finance, can now be proven to be fairer across age, gender, race, ethnicity, religion, disability, sexual orientation, sexual identity, education, national origin, or any other distinguishing characteristic or trait. In Optimization Hierarchy for Fair Statistical Decision Problems, Aswani and Olfat push data-driven decision-making closer to the promise of unbiased accuracy for an inclusive, fairer future.